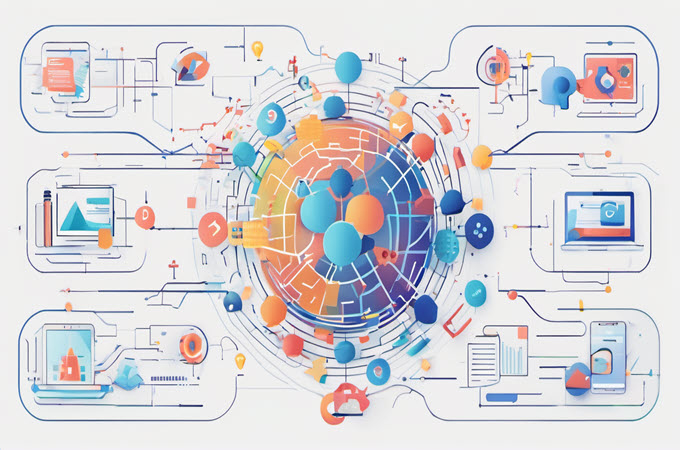
Multimodal AI, integrating text, images, audio, and video, nuances technology with perceptions akin to human cognition. Pivotal for self-driving cars to responsive virtual assistants, it stands at the forefront of next-gen innovations. Here, we dissect multimodal AI’s operational essence and survey its transformative strides across sectors, prepping you for the impending AI evolution without overwhelming you with complexities.
Key Takeaways
- Multimodal AI encompasses advanced AI systems capable of processing and integrating multiple types of data—text, images, audio, video—to simulate human perception and improve contextual understanding for enhanced decision-making.
- The architecture of multimodal AI models involves specialized neural networks for processing various data inputs, fusion techniques to merge modalities, and sophisticated output generation for accurate, comprehensive responses that can adapt to multimodal interaction.
- While Multimodal Artificial Intelligence has promising applications across sectors—from healthcare, customer service, and education to content creation—the deployment faces challenges such as data privacy, security, bias mitigation, and ensuring fairness.
Understanding Multimodal AI
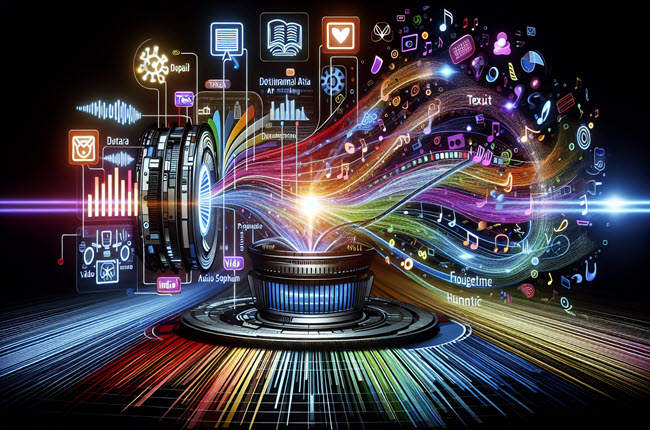
Multimodal AI refers to an advanced form of artificial intelligence that thrives on a myriad of data types, including:
- Text
- Images
- Audio
- Video
This versatility allows multimodal AI to simulate human perception in a way that was once the stuff of imagination. These systems take in the world through a variety of sensory data, much like we do, and their ability to process this information holistically enables them to grasp context and nuance far beyond that of their unimodal predecessors.
An introduction to multimodal programs’ unique appeal from its superior reasoning, problem-solving, and learning capabilities. It’s not just about making sense of different types of data; it’s about weaving these strands together to form a rich tapestry of understanding that leads to improved decision-making processes. With each modality providing a unique perspective, multimodal AI offers a more complex, nuanced view of the world—a true game-changer in the realm of artificial intelligence.
Defining Modality in AI
Within the extensive realm of AI, the term “modality” refers to the diverse types of data that an AI system can handle. These aren’t just limited to the text on a screen or the spoken word but encompass the entire spectrum from images captured by a camera to the inflections in a person’s voice. Multimodal AI, therefore, is like a polyglot, fluent in the language of pixels, phonemes, and prose. This fluency allows it to engage with the world in a manner that’s closer to human interaction than ever before.
One may wonder, why is this significant? In a world where communication is not just about what is said, but how it’s said, the ability of AI to understand these different modes is invaluable. It breaks down barriers between humans and machines, making the latter more intuitive, responsive, and, ultimately, more useful to us in our daily lives.
The Interplay of Different Modalities
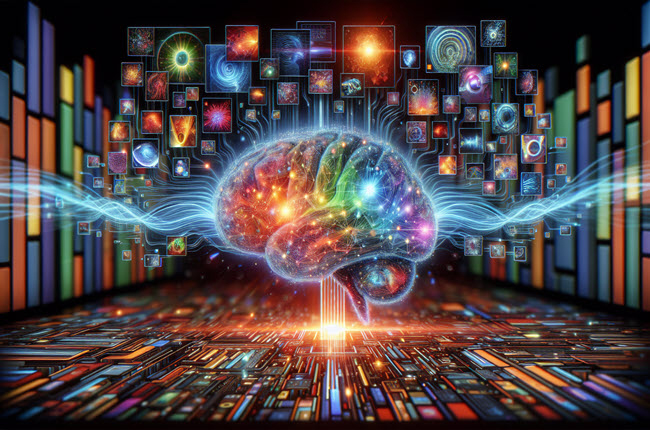
Consider how you comprehend a news story: you don’t just read the text; you also interpret images, analyze the reporter’s tone, and perhaps even read the accompanying video captions. What is Multimodal AI? It mirrors this process, enhancing their intuitiveness and dynamic capabilities by integrating various types of data. Imagine Google’s Lumiere, a model capable of transforming text into video or images into a narrative—this is the power of multimodal AI.
However, this interplay is not without its challenges. Aligning data across different modalities to represent the same event in time and space is a complex task. But when done correctly, it allows AI to build a richer understanding of the world around it, paving the way for applications such as:
- Self-driving cars that can understand and respond to what’s around them.
- Robots that can communicate with people in a friendly and easy-to-understand manner.
- Virtual helpers that can get what you’re saying and talk back to you.
- Houses that are clever enough to know what we want and make it happen.
These applications were previously thought to be the domain of humans alone.
Advancements in Multimodal Learning
Advancing into the future, Multimodal artificial intelligence remains unrelenting in its pursuit of advancements. The field is buzzing with the promise of integrating an even wider variety of data types, enhancing not just the intuitiveness of AI but also its accuracy and efficiency. With 2024 on the horizon, we’re witnessing the rise of entities like the Large Language and Vision Assistant, poised to take multimodal learning to new heights.
The interdisciplinary nature of emerging multimodal AI models will offer seamless integration and processing of tasks related to natural language, computer vision, and video data. This isn’t just an incremental step; it’s a leap towards an AI that can understand our world and its complexities as well as any human, making it an invaluable partner in our quest for knowledge and progress.
The Architecture of Multimodal Models
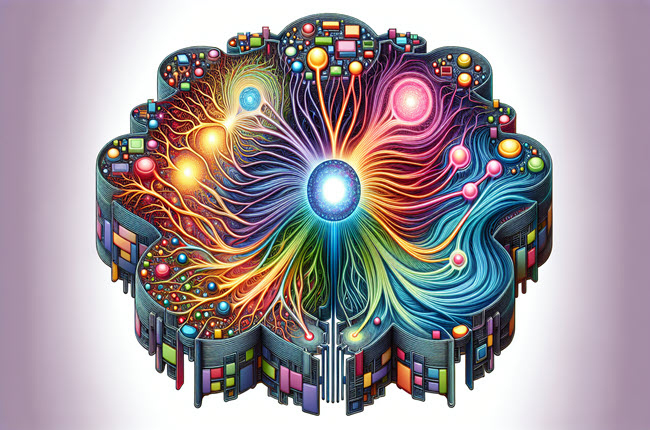
Rather than being a monolithic entity, the introduction to multimodal AI includes a meticulously coordinated ensemble of neural networks. Each network in a multimodal model is fine-tuned to process a specific type of data—be it images, text, video, or audio. This specialization enables the system to handle the nuances and intricacies of diverse data sources, creating a harmonious blend that informs more sophisticated outputs. The architecture of these systems is a marvel of modern engineering, consisting of input, fusion, and output modules that work in concert to interpret and respond to a multitude of stimuli.
Within this architecture, deep learning models play a pivotal role. They process each input with dedicated neural networks before these features are merged using a fusion network. A classifier then steps in, making predictions that guide the AI’s decisions. By incorporating capabilities from fields like natural language processing and computer vision, multimodal AI systems can create context-aware responses that are as complex as they are insightful.
Input Processing: Handling Various Data Types
The magic of multimodal AI commences at the outset with input processing. Dedicated encoders tailored for each data type transform inputs such as audio and visuals into a common feature vector representation. This is where the model begins to understand the various prompts, whether they’re written, verbal, or visual, and starts constructing a detailed response. But input processing isn’t just about handling one type of data at a time; it’s about the capability to process mixed inputs, like combining videos with images, which can present challenges for maintaining coherence.
For tasks involving complex sequences, such as lengthy videos, the AI must manage intricate dependencies between actions and maintain a coherent narrative throughout. This requires a level of sophistication that goes beyond simple data processing, demanding a deep understanding of how different types of information relate to each other and the context in which they exist.
Fusion Techniques: Merging Modalities
Following data processing, the subsequent step involves fusion. This is where the magic really happens, as individually processed modality features are woven together into cohesive representations. Fusion techniques such as concatenation and attention mechanisms are akin to an artist blending colors on a canvas, allowing the AI to perceive a fuller picture of the situation at hand. There are multiple ways to achieve this blend—
- Early fusion
- Intermediate fusion
- Late fusion
- Hybrid approaches
Each with its own merits and suited for different applications, the five modes provide a versatile approach.
A crucial aspect of fusion is understanding the semantic relationships between modalities. For example, what does the tone of a person’s voice convey when paired with their facial expression in an image? Getting this right is essential to creating effective representations that the AI can use to make informed decisions. The ongoing progression in the field aims to create models that integrate various data types more intuitively and versatilely, expanding the potential applications of multimodal artificial intelligence.
Output Generation: Creating Comprehensive Responses
Upon fusing the data into a consolidated representation, the multimodal model stands prepared to generate outputs. Drawing from the rich, synthesized data, it produces accurate and relevant responses. A classifier network takes this fused representation and, based on it, makes decisions or predictions. Meanwhile, a decision network, tailored to specific tasks, processes this information to form comprehensive responses. Systems like GPT-4V demonstrate multimodal models’ capabilities by interpreting text and images, producing outputs in multiple AI-generated voices and tackling complex interactive tasks.
Yet, developing accurate multimodal AI assistants is fraught with challenges. These systems must handle complex queries involving visual content and generate sequential responses that cater to various visual tasks. The decision networks are integral to this process, ensuring that the output is not only accurate but also meets the specific demands of the task, all while maintaining the system’s reliability and trustworthiness.
Practical Applications of Multimodal AI
The diverse sectors’ practical applications of multimodal AI truly attest to its power. Here are some examples:
- In healthcare, it is transforming patient care with more accurate diagnoses and personalized treatment plans, thanks to the analysis of medical images, patient records, and even vocal biomarkers.
- Customer service experiences are being redefined through AI-powered chatbots that can interpret text, voice, and facial expressions, leading to smarter home technologies and innovative e-commerce solutions.
- The automotive industry is not far behind, utilizing this technology to enhance in-car experiences with advanced navigation and speech recognition capabilities.
Furthermore, the media and entertainment realm is witnessing a seismic shift in content creation, recommendation systems, and personalized advertising, all driven by multimodal AI analysis.
Robotics, too, is reaping the benefits of multimodal perception, enabling robots to navigate intricate environments and understand human intentions, thereby fostering more natural and effective human-robot interactions.
Enhancing User Experience with Multimodal Interfaces
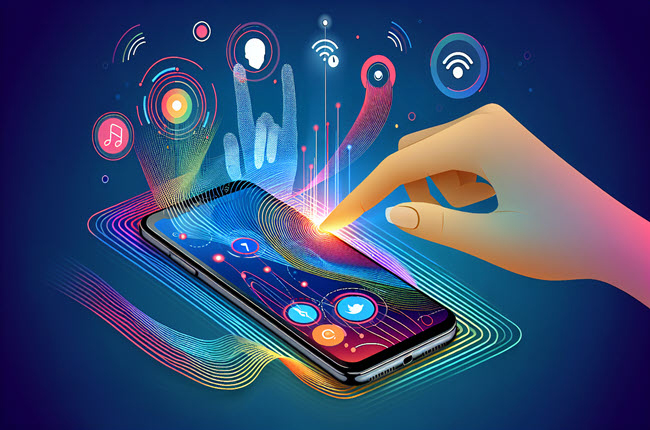
User experiences are being transformed by incorporating and introducing multimodal AI in app design, which facilitates a range of interaction styles. Voice commands, touchscreen gestures, and motion detection are all part of the multimodal interface toolkit, allowing personalized interactions tailored to each user’s preferences. These interfaces are designed with the user at the center, incorporating accessibility features and feedback mechanisms to ensure interactions are as intuitive as they are barrier-free.
For instance, augmented reality gaming offers an unprecedented level of engagement by combining audio, visual, and motion interactions via multimodal AI. Smart home speakers from industry giants are another prime example, showcasing the versatility of multimodal AI in analyzing content and responding to a wide array of tasks. However, challenges such as anthropomorphism in AI systems can significantly influence user engagement and the effectiveness of the service delivered.
Moreover, maintaining high quality and adaptability in conversational AI systems is a continuous challenge due to evolving user expectations.
Innovations in Content Creation and Analysis
What is Multimodal AI? It has revolutionized content creation, flooding our lives with a multitude of digital and paper-based multimodal texts, ranging from films and digital narratives to picture books and graphic novels. Live multimodal texts, such as dance and performance, add an interactive dimension to content, enriching the audience’s experience. Tools like Runway Gen-2 exemplify the capabilities of current content creation platforms, supporting the generation and editing of video content from diverse inputs.
Multimodal translation is pushing the boundaries of conventional translation, whether it’s translating content between different modalities or from one language to another, offering new perspectives on how we create and consume multimodal text. This innovation is not just transforming the media landscape but is also shaping new ways for individuals and businesses to communicate and connect with their audiences.
The Role of Multimodal AI in Education
Multimodal artificial intelligence is also making considerable advancements in the field of education. By creating interactive experiences that incorporate augmented and virtual reality, learning has become more engaging and personalized than ever before. Customizable learning materials powered by multimodal AI cater to individual student preferences and improve accessibility, especially for students with disabilities. Furthermore, educational technologies are now able to automate the evaluation of student work across different types of projects, providing immediate and insightful feedback.
Tools powered by multimodal AI can recognize and generate various data forms relevant to education, such as:
- Text
- Images
- Videos
- Audio
This not only enriches the educational content but also ensures that learning materials, such as the Routledge Handbook, are adaptable and inclusive, meeting the diverse needs of today’s student body through the use of a companion website.
Multimodal Tools for Learning
Immersive augmented and virtual reality environments, introduction to multimodal AI, have revolutionized the domain of digital education. These tools go beyond traditional teaching methods, offering students a chance to engage with educational content in new and exciting ways. Additionally, the real-time translation of educational materials facilitated by multimodal AI has broken down language barriers, promoting access and cross-linguistic learning.
The Meta ImageBind model is a testament to the potential of multimodal AI in education, processing text, audio, and visual data to aid in the creation of enriched content. These tools not only enhance the learning experience but also promote the development of communication skills by enabling dynamic interactions with technology across various modalities.
Fostering Multimodal Communication Skills
As digital communication flourishes, mastering various modes such as written language, visuals, audio, and spatial design is becoming increasingly imperative. Multimodal texts, which combine these elements, offer richer and more nuanced ways to convey meaning. Visual elements in communication can include a vast array of imagery, from still photos to moving videos, each carrying its own symbolism and cultural connotations. Written communication is not just about the words on a page but also involves the conventions of language, grammar, and genres that inform how ideas are expressed, as well as the text description that helps in understanding the context.
Oral communication adds another layer, encompassing mood, emotion, and elements such as speed, volume, and intonation. Also, multimodal artificial intelligence gestural communication, through body language and facial expressions, can convey meaning just as effectively as words. Spatial design in communication involves the thoughtful arrangement of elements within a space to communicate a message. Design and layout guide recipients through complex texts, while interactive meaning considers the social setting and the interplay between the audience and the subject.
To thrive in today’s world, educational institutions must emphasize teaching students these multimodal communication skills, with multimodal AI serving as a powerful tool to enhance and expand the learning experience. An associate professor, for example, can utilize this technology to create more engaging and effective lessons.
Challenges and Considerations in Multimodal AI Deployment
Even with its transformative potential, the implementation of multimodal AI comes with its set of challenges. Executing complex tasks that span different modalities can impact the efficiency and effectiveness of AI systems. Privacy concerns present significant issues, as these systems handle diverse and sensitive data types, from personal identifiers to financial details.
Ethical considerations around AI bias and discrimination are vital, as these issues affect fairness and representation across various demographic groups.
Addressing Data Privacy and Security
The extensive personal information handled by multimodal AI systems calls for rigorous data protection safeguards. The diverse data types managed by these systems make them attractive targets for cyber attacks, which could lead to exposure and compromise of sensitive personal data. To combat these threats, multimodal artificial intelligence requires robust, multi-layered security strategies that include both conventional measures and specialized approaches like adversarial training.
Localizing AI model operations on user devices might offer a way to mitigate some privacy and security concerns by minimizing data exposure to potential attackers
Mitigating Bias and Ensuring Fairness
A particularly tricky challenge in multimodal AI is the likelihood of error and bias amplification due to synchronized attacks on various data types within the model. Ensuring representation and fairness in multimodal AI is an ongoing process that requires continuous monitoring and improvement.
This includes the development of strategies to identify and mitigate biases, ensuring that AI systems serve all users equitably and without discrimination.
Navigating the Future of Multimodal AI
Looking ahead, multimodal AI is poised to become increasingly refined, comprehending intricate user needs, emotions, and contexts with enhanced depth and accuracy. Personalization will be a cornerstone of future multimodal experiences, with systems adapting interactions to individual user preferences across devices and platforms. Advanced AI assistants will leverage multimodal inputs to offer empathetic and human-like interactions, transforming the fabric of everyday life.
Businesses, too, will need to adapt to harness the benefits of multimodal programs, creating more immersive and engaging customer experiences and redefining service standards across industries. The competitive advantage in the future will lie with those who can integrate complex data sets across various modalities into their decision-making processes. However, the adoption of multmodal programs powered by AI brings societal challenges, necessitating regulations to manage impacts on employment, privacy, and equitable access to technology.
Predicting Trends and Innovations
With an anticipated growth from $1 billion in 2023 to $4.5 billion by 2028, the global market for multimodal artificial intelligence is on a rising path, signaling a boost in its adoption across industries. Small language models are emerging as both capable and efficient, offering cost-effective AI applications that comply with legal and regulatory standards.
Open source developments are democratizing the creation of multimodal AI models, enabling them to potentially outperform larger proprietary models in a variety of benchmarks.
Preparing for a Multimodal World
For businesses to prosper in a multimodal world, they need to:
- Keep abreast of AI advancements
- Fine-tune their strategies
- Incorporate AI training into their operations
- Cultivate a data-centric culture
Integrating smaller, more efficient models and applying AI tools to enhance services will be crucial for adaptation.
Companies should also focus on developing proprietary data pipelines, which allow for the customization of models to specific organizational needs, ensuring they’re well-prepared for a future shaped by multimodal AI.
Summary
As we’ve looked into it, this isn’t just a fancy tech upgrade; it changes how we communicate with machines and each other. These systems can handle lots of different kinds of info, opening up new possibilities in health, school, helping customers, and more. There are problems, like privacy worries and making sure it’s fair, but there’s also a chance to make things better. We’re right on the edge of this big change with multimodal tech, and there’s a lot of good that could come from it.
Introduction to multimodal programs means adapting to technology that understands us very well. It’s a chance to make a future where AI helps us more and brings us closer to the digital world. So, let’s get moving! Businesses, developers, and the general public should all engage, learn from it, and help improve it for everyone’s benefit.
Frequently Asked Questions
What is the difference between generative and multimodal AI?
The difference between generative and multimodal AI is that generative AI creates new content while multimodal AI can handle multiple types of data simultaneously, such as text and images, to produce various forms of output. This allows for a more comprehensive understanding and generation of content across different modalities.
What exactly is multimodal AI?
Multimodal AI refers to AI systems that can process multiple types of data inputs to simulate human perception and enhance decision-making capabilities. It integrates text, images, audio, and video to produce nuanced outputs.
What are some practical applications of multimodal AI?
Multimodal AI has diverse practical applications, such as enhancing healthcare diagnoses and treatment plans, improving customer service with AI-powered chatbots, upgrading in-car experiences, transforming content creation in media and entertainment, and enabling more natural interactions in robotics.
What challenges does multimodal AI face?
Multimodal AI faces challenges such as integrating different modalities, addressing privacy and security concerns, and mitigating AI bias to ensure fairness and equitable treatment. These challenges require careful consideration and ongoing efforts to ensure the effective and responsible development of multimodal AI.
How can businesses prepare for the future of multimodal AI?
To prepare for the future of multimodal AI, businesses should stay updated on AI advancements, refine their strategies, foster a data-driven culture, integrate efficient AI models, and develop proprietary data pipelines to customize AI applications to their specific needs. These steps will help businesses adapt and thrive in the evolving AI landscape.
Leave a Comment